Climate Data Science
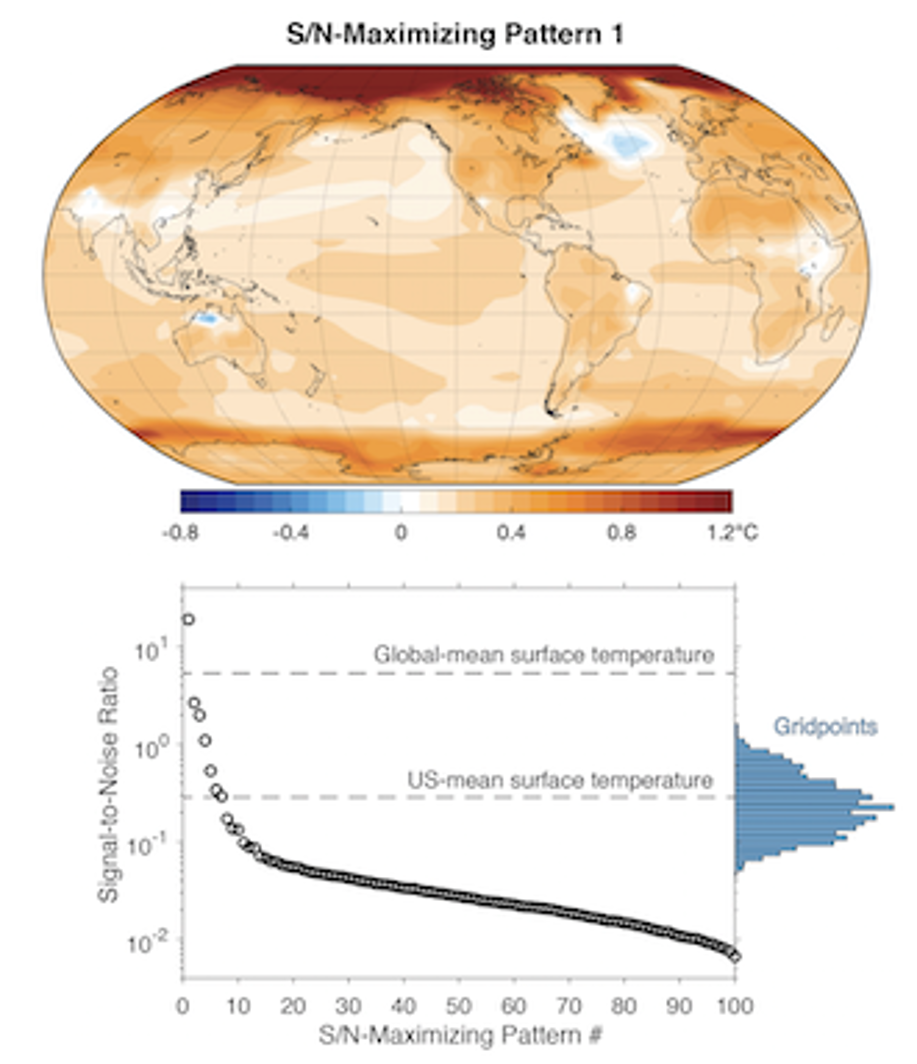
Separating the forced and unforced components of climate change is critical challenge in climate science, as it is a necessary step in understanding the causes of observed changes and in improving projections of future changes in regional climate. We develop and use pattern recognition methods to distinguish between forced and unforced components of global climate changes. In particular, our work has led to the development of low-frequency component analysis (LFCA; external page Wills et al. 2018), a specific case of linear discriminant analysis for isolating slow variations in spatiotemporal data, as well as a number of new applications of signal-to-noise maximizing pattern (S/NP) analysis (external page Wills et al. 2020). external page
In external page Wills et al. 2020, we show that pattern recognition methods such as LFCA and S/NP analysis help to separate the forced and unforced components of climate change with up to ten times fewer ensemble members than traditional methods, with LFCA even allowing an approximation of the forced and unforced components of observed changes. This helps to evaluate climate models against observations by first removing known modes of variablity such as El Niño from observations, for example showing that observed temperatures in the eastern tropical Pacific have increased less than in other parts of the tropics (external page Wills et al. 2020), a result that is not easily reproduced in climate models (see also external page Wills et al. 2022). Similarly, application of these methods to observations have been used to understand contributions to observed sea-ice variability and change (external page Dörr et al. 2023; external page Bonan et al. 2024). These pattern recognition methods also help identify hard to detect climate responses within climate models, such as changes in the jet stream over the North Atlantic and a reduction in rainfall over the U.S. Southwest in the years following tropical volcanic eruptions (external page Wills et al. 2020).
We are now co-organizing (together will collaborators at NCAR, UCLA, LLNL, and the University of Leipzig) a large community effort called the Forced Component Estimation Statistical Method Intercomparison Project (ForceSMIP), which aims to evaluate (using large ensembles of climate models) many different types of methods for estimating the forced climate response from individual realizations, including both novel machine learning methods and simple linear methods, and then to apply these methods to observations.